Protein-protein interactions (PPIs) are fundamental processes in biology. As building blocks, they help researchers understand biological functions and mechanisms, which in turn shapes our understanding of diseases. Unfortunately, there is no exhaustive list of PPIs - the space of all potential PPIs is large and it is costly to verify them. In a recent seminar at the Center for Network Science, István Kovács showed how new network science methods can be used to predict likely PPIs quickly and much more accurately than before.
As with any relational data, PPIs have a natural interpretation as a network: two proteins are connected if they interact with each other. Previous researchers have translated the problem of predicting PPIs into a question about link prediction. Given a network with missing edges, in a classical link prediction task, the aim is to identify pairs of nodes which are likely to be connected from other information available in the network. In the biological case, we are talking about actual missing links.
Most link prediction methods are applied to social networks and their heuristics reflect this background: I may predict that two colleagues will become friends if they have many mutual friends in common. In other words, the similarity of two nodes, used implicitly to suggest that they are likely candidates for linking, derives from the similarity of their connectivity. Unfortunately, this philosophy for link prediction performs very poorly when predicting PPIs. In Figure A we share István’s presentation of the theorized and actual relationship between the similarity and connection probability.

Figure A: Connectivity similarity was theorized to predict PPIs, but the data shows the opposite: two proteins sharing many common neighbors are less likely to interact themselves.
István came up with a fascinating solution to this problem. By observing case studies, he noted the physical shape of a protein limits its potential interactions. Proteins should hence not be expected to interact with other proteins sharing many of the same neighbors; that would be like trying to connect two electrical plugs instead of a plug with a socket. Rather the insight is that, given a protein A, the neighbors of a highly similar protein B are the best candidates for a missing link with A. In network terms, A and B are more likely to connect if they are connected by many paths of length 3. The data support this finding, visualized in Figure B.
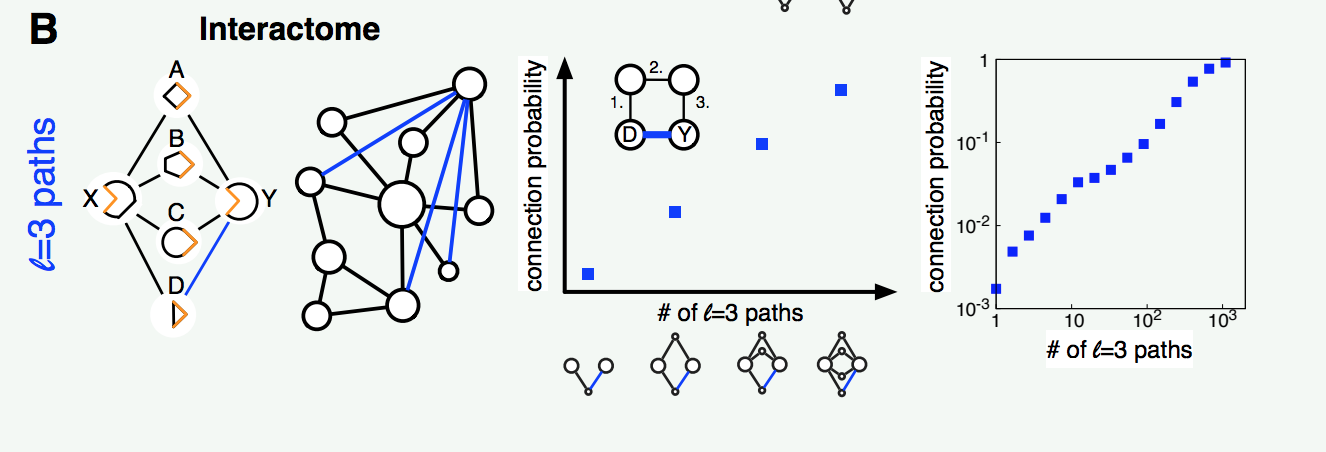
Figure B: Paths of length three predict missing links very well.
István’s findings are the result of close cooperation with biologists and a deep understanding of the topology and function of the nodes and edges of the PPI network. The applications extend beyond predicting previously unknown PPIs: these new insights have found use in the evaluation of drugs and virus-host interactions. From a network science perspective, this research underlines that we cannot always translate methods between networks from different domains. Indeed, in this case, the overeager application of a social networks analogy to a biological network leads to link predictions which perform worse than random guessing. István’s cutting-edge research is on the forefront of the field and not yet published.
We were also excited to catch a preview of István’s ongoing work on infection spreading. We look forward to hearing more.
Blog post by Johannes Wachs