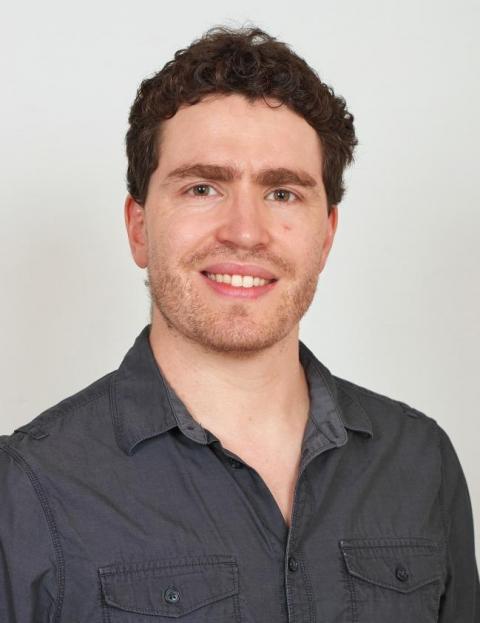
Weighted networks provide meaningful information for the structure of complex systems where interacting entities are connected with links weighted by the strength of their interaction. In his recent presentation, Gerardo Iñiguez González, Assistant Professor at the Department of Network and Data Science at CEU, shared his research about threshold driven contagion on weighted networks.
Our social behaviors are likely to be influenced by the decisions of our family and friends. If the fraction of our neighbors that do a certain decision exceeds a threshold, then we tend to have the same behavior. We are easily influenced if the threshold is small. In most of the previous studies, social networks were considered simplistically with the assumption that all links in the network contribute the same to social influence. However, some people in real networks are more important than others, such as family and close friends, having more influence in our decision making. In order to have a more accurate representation of real-world dynamics and study social contagion properly, weights should be considered in the network.
The simplest possible case is a k-regular random network with a certain bimodal weight distribution, where strong weight characterizes close relations and weak weight represents weak relations such as Facebook friends. For a node in the network, if the fraction of weights associated with the neighbors that have already adopted among all social neighbors exceeds the threshold, then the node adopt the same behavior. Figure 1 illustrates the probability and speed of cascades behavior in the weighted network. As the threshold increases, people are more reluctant to adopt. When the time of the cascade happens is small, the speed of the contagion is fast. The cascade time increases as the threshold increases.
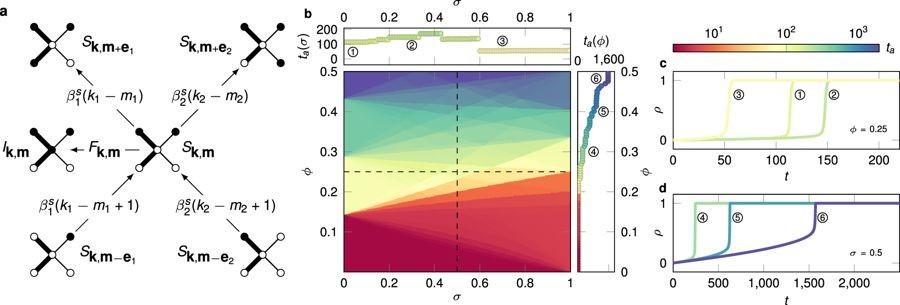
Figure 1. Threshold driven contagion and cascade evolution on weighted networks.
The effect of weight is non-monotonous in terms of the cascade time compared to the unweighted case. Let denote the time of cascade emergence relative to the unweighted case with the same threshold value. When the relative time is negative, the cascade in the weighted case as the blue area shows takes longer than the unweighted case. When it is positive, the cascade in the weighted case evolves faster as the red area shows. Figure 2 shows the non-monotonous spreading behavior and partial cascades as the main consequences of weight heterogeneities in threshold driven contagion.
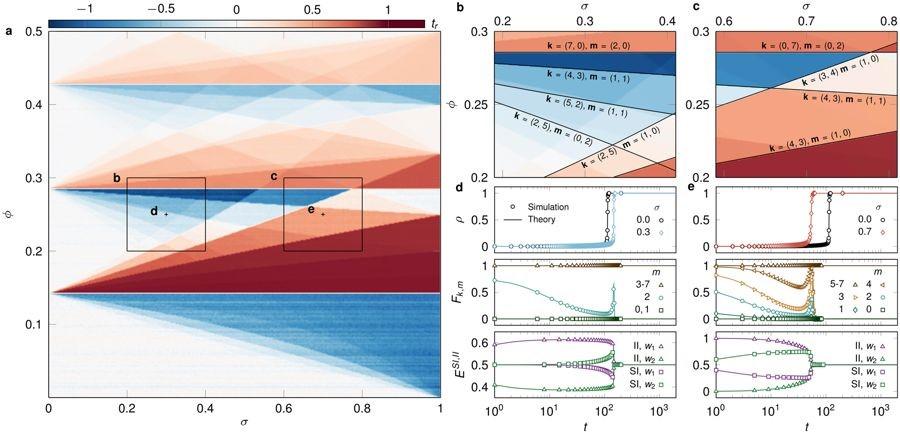
Figure 2. Relative time of threshold driven cascades on weighted networks.
The effect of structural heterogeneities are studied when moving from a k-regular network to a network with a broad degree distribution (in this case the scale-free network), and skewed bimodal weight distribution. Data-driven simulations are implemented on a signed network of Wikipedia editors. As the relative time of cascade emergence simulation shows, weights slow down the contagion in some areas while in other areas speed up the process. Prof. Iñiguez clarified the network originally was directed and they only took links which are mutual, responding to a comment by Prof. János Kertész that the network was directed. The interpretation of negative weights for the links of social contagion still remains an open question.
The effects of weight heterogeneities are studied by considering a k-regular (k=7) configuration network with heterogeneously distributed degrees and weights. Another data-driven simulation is implemented in a large empirical weighted social network, the mobile call network, where a link appears when two people call each other mutually in a certain period of time and weights are proportional to the number of calls between individuals. This network has around 6.2 million nodes and 16 million edges, with a lognormal degree and weight distribution. Figure 3 shows threshold contagion on heterogeneous synthetic and real networks.
Overall, the networks are explored with increasing complexity, from configuration-model networks with bimodal or lognormal weights, to real-world networks with a broad degree and weight distributions. Threshold driven contagion depends non-monotonously on weight heterogeneity, creating slow or fast cascades relative to the equivalent unweighted spreading process. Finally, Prof. Iñiguez proposed possible future research directions about multiplexity on link differences, temporal networks and product competition in social contagion.
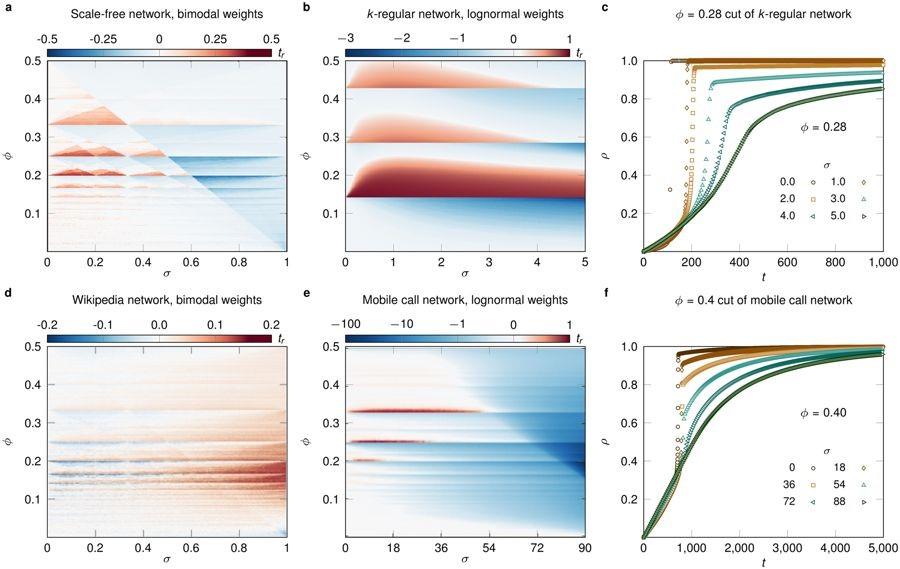
Figure 3. Threshold contagion on heterogeneous synthetic and real networks
Reference: Samuel Unicomb, Gerardo Iñiguez, and Márton Karsai. "Threshold driven contagion on weighted networks." Scientific reports 8.1 (2018): 3094.
https://www.nature.com/articles/s41598-018-21261-9
Blog post by Hao Cui